Just because it’s brown, doesn’t mean it’s chocolate
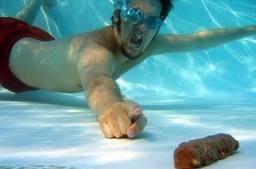
This blog entry comes courtesy of John Woodslave who posted the link on my Facebook page.
Martin Bland’s “How to Upset the Statistical Referee” should be mandatory reading for all researchers. It’s short, gets to the point and, if everyone paid ACTUAL attention to it, would kill this blog.
For most guys, building muscle is the goal. It’s not about building lean mass; it’s muscle-building. Getting mono usually results in an increase in lean mass, but it’s all in your spleen. No one’s buying that e-book.
Quantifying muscle building, however, is difficult. For one, hydration plays a significant role in muscle size, so even scanning cross-sectional methods can be tricky, but in my opinion is still the best way of quantifying muscle growth in a meaningful fashion. Protein quantification methods however, remain one of the most-used proxy measurements of muscle growth. What’s a proxy measurement, you ask? A proxy measurement is basically an indirect measurement. Sometimes, you can’t measure something directly. The only way to absolutely know what soup is in a can is to open it. But it’s rather impractical to do this if you have several cans of different soup and you want cream of mushroom. Fortunately, soup cans come with labels that are generally reliable. The label is a proxy measurement for the soup.
Proxies can come in good and poor varieties though. Using the label on a soup can is a pretty good proxy for what soup is in the can. Using how brown something is as a proxy for how much chocolate is in a tubular-shaped mass is probably less than good. All proxies require assumptions. You assume that the soup company correctly labels their soup cans correctly almost all of the time to make the leap of faith that when you open a can of soup that’s labelled, “Tomato soup”, it’s not going to be chicken broth.
Protein quantification is what I would consider a moderately useful proxy for muscle building. It requires us to make some assumptions that may or may not actually hold. When most people think about protein in the body, they think about muscle. But the reality is that everything structural in your body that isn’t water, fat or calcium is made primarily of protein, specifically collagen. This includes your heart, lungs, guts, liver, blood vessels, nerves, skin and nails and bones. Measuring protein synthesis and breakdown does not differentiate between protein that’s laid down or broken down in muscle and protein that’s laid or broken down in every other tissue. However, the assumption is made that changes in protein balance reflect changes in primarily in muscle.
Post-workout nutrition remains one of the most controversial topics when it comes to muscle building. How much protein, when to take it, whether it’s necessary; all questions that we don’t have great answers to yet.
Moore DR et al. Daytime pattern of post-exercise protein intake affects whole-body protein turnover in resistance-trained males. Nutrition and Metabolism, 9:91, 2012.
So, in an attempt to get at how much and when, these researchers took 24 men who lifted weights 4-6 times per week. Everyone got a DEXA scan and had their 1RM measured for leg extension. The subjects got a standardized diet for 72 hours before the trial (45kcal/kg of fat free mass, 1.5g of protein/kg, 4g of carbs/kg). Subjects were told to avoid training during this 72 hour period.
On the trial date, subjects fasted for 10 hours overnight. Baseline urine samples were taken. They then did two warm-up sets (60% and 70% 1RM) and the 4 sets of 10 reps at 80% 1RM with 3 minutes between sets. The subjects then got one of three post-workout protocols (all protocols were 12 hours in duration):
1) PULSEd feeding: 10g of protein every 1.5 hours
2) INTermediate feeding: 20g of protein every 4 hours
3) BOLUS feeding: 40g of protein every 6 hours
All urine was collected over the 12 hours. The first protein drink contained radio labelled glycine so that the ammonia could be measured to produce a measurement of whole-body protein turnover (synthesis – breakdown). The authors also adjusted the turnover numbers for total body mass as well as fat and bone-free body mass (which leaves muscle, tendon, guts, hearts, skin, eyes…)
Statistics:
Data was compared between the groups using a one-way repeated measures ANOVA with post-hoc Student Newman Keuls comparison. The authors explicitly state that, “Statistical significance was established at P<0.05…" Effect sizes were also calculated because the researcher didn't want to miss "possible subtle differences" in nitrogen balance. More on this later. Results
The results of this study are reported in a very interesting way.
The authors report first that whole-body NITROGEN turnover, when adjusted for body mass was greater for the PULSE group than the BOLUS group (p<0.05). However, the authors failed to find evidence for a statistical difference between any of the groups in protein balance when they ran the ANOVA. The authors keep referring to differences "trending" towards statistical significance, and "likely moderate and small positive effects…" From an effect size point of view, none of the effect sizes yielded statistically significant p-values, and from an interpretation point of view of the actual effect sizes, the confidence intervals were so wide, that the range of plausible values includes trivial effect sizes. The authors however, provided explicit “interpretations” stating that despite p-values between 0.15 and 0.46, that small to moderate increases were likely or possible. The authors concluded in this paper, “…whole-body protein balance tended to be greatest with moderate 20g feedings every 3 hours, which may have implications for individuals aiming to enhance whole-body anabolism including lean body mass accrual with training,” and that the pattern of feeding, not just the amount of protein consumed, should be taking into consideration. Discussion
There are two perspectives I want to talk about here with regards to this study: 1) the interpretation of the data from a deduction point of view, and 2) the interpretation of the data from a statistical point of view. Both paths, however, lead to the same destination.
Protein turnover, in this study, is defined as protein synthesis minus protein breakdown. A higher turnover number in one group compared to another, therefore, would mean that either more protein is being synthesized or that less protein is being broken down, which poses an interesting deduction conundrum because I’m not entirely sure it’s possible to tell which scenario applies. In the former, you could draw the indirect conclusion that at least some of the extra “retained” protein is being incorporated into muscle, thus resulting in muscle building. But in the latter, while the dietary pattern could be responsible for preventing additional protein breakdown, doesn’t necessarily result in actual muscle growth. Therefore, I’m not entirely sure that one can actually state that a higher protein balance number can be associated with higher anabolism; only that it’s not catabolism, because the number isn’t negative.
So while protein turnover is an interesting proxy measurement, you can see how it’s a little more like using brown as a proxy for chocolate than it is like using a soup can label as a proxy for soup.
The statistical interpretation, however, is much more interesting.
The interpretation of p-values is very much like interpreting a pregnancy test. One is either pregnant, or not pregnant. You can’t _approach_ pregnancy. You can’t be almost pregnant, kinda pregnant, sort of pregnant, trend towards pregnancy. Similarly, a p-value is either statistically significant, or it’s not. If you define, prior to the experiment, that you will only consider p-values less than or equal to 0.05 as statistically significant, then the resultant p-value either meets the criteria or it doesn’t.
Remember that a p-value is the probability of observing the measured difference between groups if the reality is that there is no meaningful difference between them. If the p-value is very low, then we infer that the groups must be fundamentally different because the likelihood of measuring that difference if they were fundamentally the same is so remote that it’s almost impossible. It’s like saying that if you flipped a coin 100 times and got 95 heads, that the chances of that happening with a fair coin are so low, that it would be reasonable to conclude that the coin is UNfair. Failing to find evidence for a statistically significant difference means that the difference you observe could be due to random chance alone, and not to an underlying fundamental difference due to the feeding pattern of protein. Everything is POSSIBLE, the role of using inferential statistics is to determine whether it’s PROBABLE.
The other interesting “sin” in this paper is the use of pairwise comparisons after the omnibus test has not yielded a significant p-value. It’s common practice to use an ANOVA to determine if any differences exist in a group of differences, and then to break it down pair by pair to see which specific differences are the statistically significant ones. We know that multiple significance tests increase the chance of finding a spurious significant p-value. The ANOVA is known as the “omnibus” test, and indirectly protects the analysis from having to adjust the p-values for multiple significance tests. However, having done the ANOVA and gotten a p-value of 0.23, the authors went on to do all the pairwise comparison tests (PULSE vs INT, PULSE vs BOLUS, INT vs BOLUS) and found one significant value (which is only reported at p<0.05). So if the omnibus test fails to yield a significant p-value, how does one interpret the contradictory signifiant value? What’s more is that the effect size of the one, probably spuriously significant p-value between PULSE and BOLUS was 0.59, which would be considered small, and not likely meaningful. And when the authors suggest that the greatest whole-body protein turnover was observed in the INT group (20g every 3 hours), the effect size was a non-significant, but possibly moderate 0.8 when compared to the BOLUS group, but only 0.42 (not only somewhere between trivial and small, but also not statistically significant) when compared to the PULSE group. In the end, this study raises an interesting question and at best, acts a pilot data for a larger trial. I am somewhat abhorred at the misuse of statistics but am impressed that a study with this many non-significant p-values could actually be considered evidence to chance dietary patterns. I would vehemently disagree with the authors’ conclusions because it cannot be ruled out that the differences they observed were not observed simply by dumb luck, though I’m a little jealous of their audacity (Don’t even get me started on how misleading the abstract reads). If we’re going to act based on “trends towards significance”, I’m pretty sure wearing purple socks on Sundays can be shown to increase protein balance too. I just don’t think it’s right to make people collect pee for 12 hours to show it.